[ad_1]
From Pixels to Insights: Understanding the Basics of Computer Vision
Computer Vision, a rapidly evolving field of Artificial Intelligence, aims to enable computers to obtain high-level understanding from digital images or videos. It involves the development of algorithms and techniques that allow machines to process and interpret visual information similar to how humans do. Computer Vision holds immense potential across various industries, including healthcare, transportation, robotics, and surveillance, among others.
At its core, computer vision focuses on transforming raw pixel data into meaningful insights and actionable information. To achieve this, it follows a series of steps involving image acquisition, preprocessing, feature extraction, and understanding.
The first step in computer vision is image acquisition, where digital images or videos are captured either by traditional cameras or more advanced imaging devices like infrared cameras or 3D scanners. These images are crucial as they form the foundation for subsequent processing and analysis.
Once the images are acquired, preprocessing techniques come into play. Preprocessing involves various operations like noise removal, image enhancement, and normalization to clean and standardize the data. This step is vital as it helps to enhance the overall quality of the images and reduce unwanted artifacts or distortions.
After preprocessing, the focus shifts to feature extraction. Feature extraction is the process of identifying distinctive patterns or attributes in the images that are relevant for further analysis. These could include edges, corners, textures, colors, or even more complex features like shapes or objects. The selection and extraction of these features play a crucial role in subsequent stages of computer vision, such as object recognition or classification.
Once the features are identified, the next step is understanding or interpretation. This stage involves the application of various algorithms and techniques for analyzing and extracting meaning from the extracted features. It could include tasks like object detection, recognition, tracking, or even more complex tasks like scene understanding or image captioning. This stage is where the real power of computer vision lies, as it enables machines to comprehend and interpret visual information with increasing accuracy.
Deep learning, a subset of machine learning, has revolutionized computer vision in recent years. Convolutional Neural Networks (CNNs) have become the backbone of many computer vision applications, as they can automatically learn and extract features from images with exceptional accuracy. CNNs have been instrumental in achieving breakthroughs in image recognition tasks, including surpassing human-level performance in image classification challenges.
Computer Vision has made significant advancements in recent years, thanks to the availability of large datasets, computational power, and advancements in algorithms. This progress has led to various real-world applications like autonomous vehicles, facial recognition, medical imaging, and augmented reality. These revolutionary applications have the potential to transform industries, save lives, and improve human experiences in unimaginable ways.
However, it is essential to acknowledge that computer vision is an ongoing field with its own set of challenges. It still struggles with issues like occlusion, lighting variations, and complex scene understanding. Researchers are continuously working towards addressing these challenges and refining algorithms to improve accuracy and robustness.
In conclusion, computer vision is a rapidly evolving field that continues to push the boundaries of what machines can achieve in terms of visual understanding. By transforming raw pixel data into actionable insights, it enables machines to perceive and interpret the visual world around us. As technology continues to advance and algorithms improve, the potential for computer vision to revolutionize industries and augment human capabilities is truly remarkable.
[ad_2]
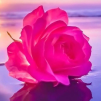