[ad_1]
Demystifying Deep Learning: The Science Behind AI Breakthroughs
Artificial Intelligence (AI) has witnessed groundbreaking advancements over the years, transforming the world we live in. One of the most significant breakthroughs in this field is deep learning, a subset of machine learning that has revolutionized AI capabilities. Deep learning algorithms are responsible for developing self-driving cars, voice assistants like Siri and Alexa, and even defeating human champions in complex games like chess and Go. But what exactly is deep learning, and how does it work?
At its core, deep learning is an artificial neural network composed of multiple layers of interconnected nodes, which are analogous to the human brain’s neurons. These networks are trained to recognize patterns and extract meaningful insights from vast amounts of data. Combining layers of nodes allows the network to learn increasingly complex representations, enabling it to make accurate predictions or classifications.
Deep learning algorithms excel in solving problems that require a high degree of abstraction, such as image and speech recognition. This is achieved through a process called gradient descent, which fine-tunes the neural network parameters by minimizing the difference between its predictions and the actual values. The network continuously adjusts its internal connections based on the feedback received during the training phase, gradually improving its accuracy.
To understand how deep learning algorithms can make groundbreaking AI breakthroughs, it is essential to delve deeper into the underlying science. One of the key components of deep learning is the artificial neuron, also known as a perceptron. It takes multiple inputs, multiplies them by respective weights, and passes them through an activation function to produce an output. The activation function is responsible for introducing non-linearity into the network, enabling it to model complex relationships between inputs and outputs.
The true power of deep learning lies in its ability to automatically learn feature representations from raw data. In traditional machine learning approaches, engineers would manually design and select features to feed into the learning algorithm. However, deep learning eliminates this need by learning the most informative features directly from the data. It autonomously extracts hierarchical representations, starting with low-level features like edges and gradually moving towards high-level abstract concepts.
Convolutional Neural Networks (CNNs) are particularly effective in image recognition tasks. These networks utilize convolutional layers to detect visual patterns, such as edges or textures, and then combine them to identify higher-level visual features. CNNs can recognize objects, localize them within an image, and even generate detailed captions describing the content.
Recurrent Neural Networks (RNNs) are adept at processing sequential data, such as speech or text. RNNs have a unique inherent property called memory, enabling them to capture temporal dependencies and extract context from past information. This makes them well-suited for tasks like speech recognition, language translation, and even generating human-like text.
One of the challenges with deep learning is the requirement for massive amounts of labeled data for training. Large datasets ensure that the neural network can learn diverse patterns and generalize well to unseen examples. However, gathering and labeling such data can be time-consuming and expensive. To overcome this, researchers have developed techniques like transfer learning, where pre-trained models are used as a starting point. These models have learned from vast amounts of data and can be fine-tuned for specific tasks, even with limited labeled data.
In conclusion, demystifying deep learning reveals the science behind AI breakthroughs, explaining how neural networks, formed by layers of interconnected nodes, can learn complex representations and perform tasks like image recognition, speech synthesis, and natural language processing. As the field of AI continues to advance, deep learning remains at the forefront, potentially unlocking more groundbreaking innovations yet to come. The future looks promising as scientists and engineers continue to explore the untapped potential of deep learning and AI.
[ad_2]
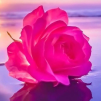