[ad_1]
Deep Learning: Revolutionizing Artificial Intelligence
Artificial Intelligence (AI) has come a long way since its inception, but there has been a significant breakthrough in recent years that has propelled it to new heights: Deep Learning. With its ability to process vast amounts of data and create complex models, Deep Learning has revolutionized the field of AI, making significant advancements in areas such as image recognition, natural language processing, and speech synthesis.
Deep Learning is a subfield of machine learning, which focuses on the development of algorithms that allow computers to learn from and make predictions or decisions based on large datasets. However, what sets Deep Learning apart is its use of artificial neural networks, loosely inspired by the biological neural networks present in the human brain.
The basic unit of a neural network is a neuron, which receives inputs, applies a mathematical operation to them, and produces an output. These neurons are organized in layers, forming a network. Deep Learning networks consist of multiple layers, with each layer responsible for extracting increasingly complex patterns from the input data. The deep structure of these networks allows them to leverage hierarchical representations, modeling more sophisticated relationships between features.
One of the most prominent applications of Deep Learning is in image recognition. Convolutional Neural Networks (CNNs), a type of Deep Learning network, have achieved remarkable accuracy in tasks such as object detection, facial recognition, and image classification. By learning from massive labeled datasets, CNNs are capable of identifying and categorizing objects with great precision.
Natural Language Processing (NLP) is another area benefiting from Deep Learning. Recurrent Neural Networks (RNNs) and Long Short-Term Memory (LSTM) networks, both variations of Deep Learning networks, have proven essential in tasks like sentiment analysis, language translation, and speech recognition. Through sequential processing and memory capabilities, these models can understand the context and meaning behind textual data, leading to more accurate language understanding and generation.
Despite its tremendous achievements, Deep Learning still faces challenges. One significant issue is the need for massive amounts of labeled data for training, which can be time-consuming, expensive, and sometimes unfeasible to obtain. Additionally, the interpretation of the internal workings of deep networks, often referred to as the “black box” problem, raises concerns about their explainability and trustworthiness.
Nonetheless, research on Deep Learning is progressing rapidly, with continued efforts focused on overcoming these challenges. Techniques like transfer learning, semi-supervised learning, and generative models aim to reduce the data requirements and tackle the interpretability problem.
The impact of Deep Learning on society is already vast, and its potential seems boundless. Industries ranging from healthcare and finance to transportation and entertainment are harnessing Deep Learning’s power to drive innovation. Self-driving cars, personalized medicine, virtual assistants, and recommendations systems are just a few examples of how Deep Learning is transforming our everyday lives.
In conclusion, Deep Learning is revolutionizing the field of Artificial Intelligence, enabling machines to analyze and understand massive amounts of data, and perform complex tasks with human-like accuracy. As research progresses, the barriers holding deep networks back will likely diminish, allowing us to unlock the full potential of AI and its applications across various domains. With Deep Learning leading the way, we are witnessing a new era in AI that holds great promise for the future.
[ad_2]
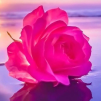